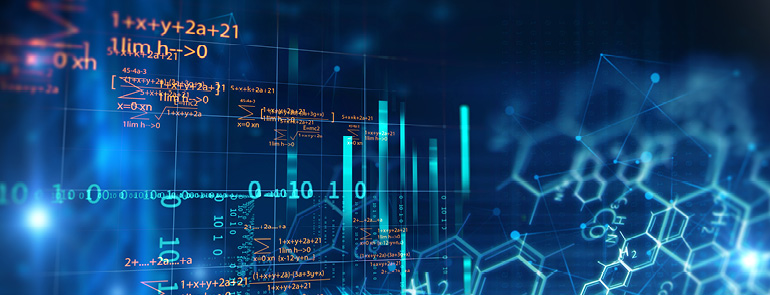
Use prior information to improve process development and control.
By Katherine Giacoletti, M.Stat., and Tara Scherder, MS Statistics, both with SynoloStats LLC
To build upon previous knowledge with new information is logical and intuitive, and we do so day in and day out: we choose the route to work that experience has shown to be the fastest and/or least stressful, we judge the veracity of news based on a historical sense of plausibility and reliability of the source, and we weigh the odds of political or sports outcomes based on past performance, to name just a few examples. These judgments and predictions are not always correct, but no one would argue that inaccuracies are always due to using prior knowledge. And most would acknowledge that not using previously gained knowledge is a mistake.
Likewise, throughout the lifecycle of a pharmaceutical process, it is clearly logical to use existing information to guide design choices and evaluate performance. We use platform knowledge to inform the design of a new process, we use past process performance to interpret new process data, and we use knowledge of measurement variability to identify and understand unusual data points, etc. If we could incorporate this type of prior knowledge into statistical models that we use for decision making, would we not choose to do so? Particularly if these models enabled more efficient development, fewer resources, and better prediction of performance? This article introduces this capability using Bayesian statistical methods, which are increasingly applied in both social and scientific applications, including pharmaceutical research, development, and manufacture.